As technology continues to advance, the integration of edge computing and AI in data centers has become increasingly vital.
Edge AI data centers offer the ability to process and analyze vast amounts of data closer to its source, resulting in reduced latency and improved real-time capabilities.
With the growing integration of AI and 5G technology, investments in edge infrastructure and AI hardware for data centers are expected to rise, benefiting not only efficiency and performance but also the overall AI market and economy.
Furthermore, the advantages of edge computing, such as lower latency, enhanced bandwidth efficiency, improved security, and increased reliability, make it a compelling solution for AI applications.
Therefore, it is crucial to explore the possibilities and challenges that arise from this convergence of edge computing and AI in data centers.
Key Takeaways
- AI-optimized hardware enhances the performance and efficiency of data centers for AI workloads, addressing the challenge of immediate responses in industries like autonomous vehicles and telemedicine.
- Integrating edge computing and AI enhances data processing capabilities, enabling real-time decision-making and reducing latency.
- Edge computing and AI improve network efficiency by processing and filtering data at the edge, optimizing bandwidth usage.
- The integration of edge computing and AI enables faster processing and analysis of data, leading to improved performance of AI applications and services.
Ai-Optimized Hardware for Enhanced Data Centers
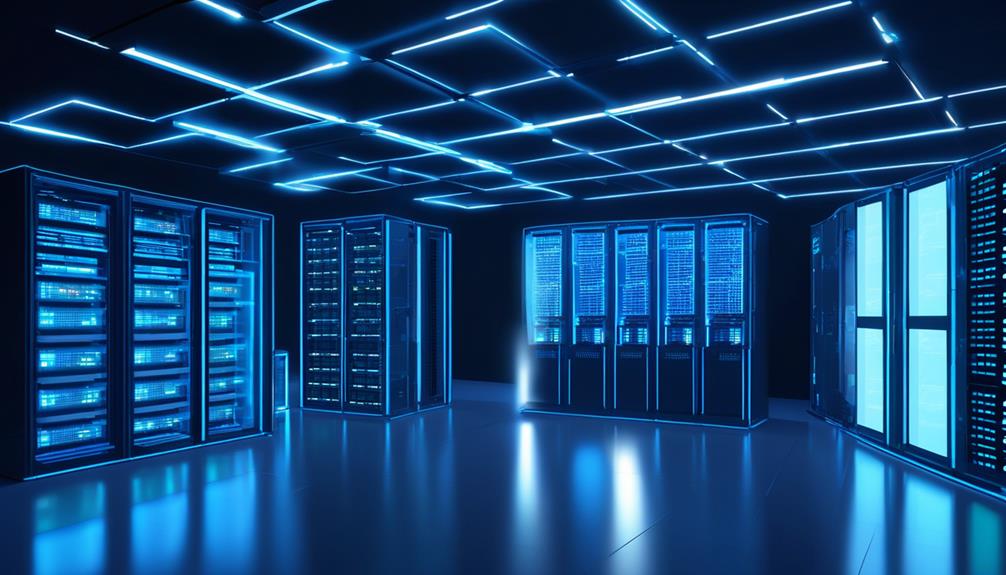
AI-optimized hardware, designed to enhance the performance and efficiency of data centers for AI workloads, plays a crucial role in facilitating faster and more accurate data processing for AI applications. These investments in hardware target core infrastructure such as servers, storage systems, and networking equipment within data centers. By optimizing these components specifically for AI workloads, organizations can maximize their computing resources and improve the overall efficiency of their data centers.
One of the key challenges in AI applications is latency, the delay between the input of data and the output of results. AI-optimized hardware addresses this challenge by reducing latency and enabling real-time processing. This is particularly important in industries such as autonomous vehicles and telemedicine, where immediate responses are critical.
Furthermore, AI-optimized hardware contributes to the deployment of AI solutions across various industries. With the increasing demand for AI applications, data centers need to be equipped with the necessary infrastructure to handle the computational requirements. AI-optimized hardware provides the necessary computing power and resources to support the complex algorithms and models used in AI applications.
Looking towards the future, the integration of AI and edge computing is a promising trend in the development of AI-optimized hardware. Edge computing brings the processing power closer to the data source, reducing latency and improving response times. This is especially beneficial for AI applications that require real-time decision-making, such as in autonomous vehicles or industrial automation.
Benefits of Integrating Edge Computing and AI
Integrating edge computing and AI brings several benefits to data centers.
Firstly, it enhances data processing capabilities, enabling real-time decision-making at the source and reducing latency.
Secondly, it improves network efficiency by processing and filtering data at the edge, resulting in optimized bandwidth usage.
These advantages contribute to the scalability, reliability, energy efficiency, security, and privacy of data centers.
Enhanced Data Processing
The integration of edge computing and AI revolutionizes data processing, enhancing the capabilities and efficiency of real-time analysis. This combination offers several benefits for data centers and AI applications:
- Improved Performance: Edge computing and AI integration enables faster processing and analysis of data, leading to improved performance of AI applications and services. This is particularly valuable in time-sensitive scenarios where quick decision-making and response times are crucial.
- Reduced Latency: By processing data at the edge, closer to the source, edge computing minimizes latency, allowing for near real-time analysis. This reduced latency enhances the speed and efficiency of data processing, enabling faster insights and actions.
- Enhanced Bandwidth Efficiency: Edge computing reduces the volume of data transmitted over networks by performing processing tasks locally. This leads to improved bandwidth efficiency, as only relevant data is sent to centralized data centers for further analysis. Consequently, it reduces network congestion and lowers operational costs.
The integration of edge computing and AI in edge data centers brings significant advancements to data processing, enabling more efficient and responsive AI applications in various industries.
Real-Time Decision Making
Real-time decision making is a key advantage of integrating edge computing and AI, enabling faster responses to data insights. By processing data locally at the edge, the latency is significantly reduced, allowing for quicker decision-making. This integration enhances efficiency and accuracy in decision-making processes, as it eliminates the need to rely solely on centralized data centers. The combination of edge computing and AI facilitates localized processing, enabling on-the-spot, autonomous decision-making. This real-time decision-making capability is particularly crucial for critical applications where immediate actions are required. The following table highlights the benefits of integrating edge computing and AI in data centers:
Benefits of Integrating Edge Computing and AI |
---|
Faster Responses to Data Insights |
Lower Latency |
On-the-Spot, Autonomous Decision-Making |
Integrating edge computing and AI enables data centers to make real-time decisions based on localized and processed data, ensuring timely actions and improved operational efficiency.
Improved Network Efficiency
Improved network efficiency is a significant advantage achieved through the integration of edge computing and AI in data centers. This integration brings forth a range of benefits that enhance the performance and reliability of network operations.
Here are three key ways in which edge computing and AI improve network efficiency:
- Reduced data transmission: Edge computing and AI integration minimize the amount of data transmitted over networks to central data centers. By processing and analyzing data locally at the edge, only relevant and actionable insights are transmitted, reducing network congestion and optimizing bandwidth efficiency.
- Lower latency: Investments in edge computing and AI result in decreased latency for real-time responses. Localized processing at edge data centers enables faster decision-making, enhancing the speed and performance of network operations.
- Enhanced security: With localized processing, sensitive data can be processed at the edge, reducing the risk of data breaches. This approach ensures better compliance with data sovereignty laws and provides better security for critical information.
Through the combined power of edge computing and AI, data centers can achieve improved network efficiency, enabling faster, more secure, and cost-effective operations.
Improving Data Center Capabilities With Edge Computing
Edge computing is a transformative solution that enhances data center capabilities for improved processing and reduced latency. By bringing AI processing closer to the data source, edge computing services enable real-time capabilities, making it a critical component in the evolution of data centers. Investments in edge computing infrastructure and hardware drive advancements in AI capabilities, integrating AI and 5G technology for more efficient processing.
SiMa.ai, for example, offers edge computing solutions that enable efficient AI processing. Their technology has been exemplified by improved video analysis on drones, showcasing the potential of edge computing in various industries. Additionally, Lenovo's TruScale for Edge and AI service, leveraging the ThinkEdge SE455 V3 server, caters to diverse deployment needs, from retail outlets to fishing boats, further expanding the reach of edge computing in data centers.
Edge computing not only improves processing capabilities but also offers several other benefits. By reducing the amount of data transmitted to the central data center, edge computing conserves network resources, optimizing bandwidth usage. This approach also enhances security by processing sensitive data closer to the source and minimizing the risk associated with transmitting data over long distances. Furthermore, edge computing enables scalability, allowing data centers to easily accommodate the growing demands of AI and IoT applications.
Leveraging AI in Edge Data Centers
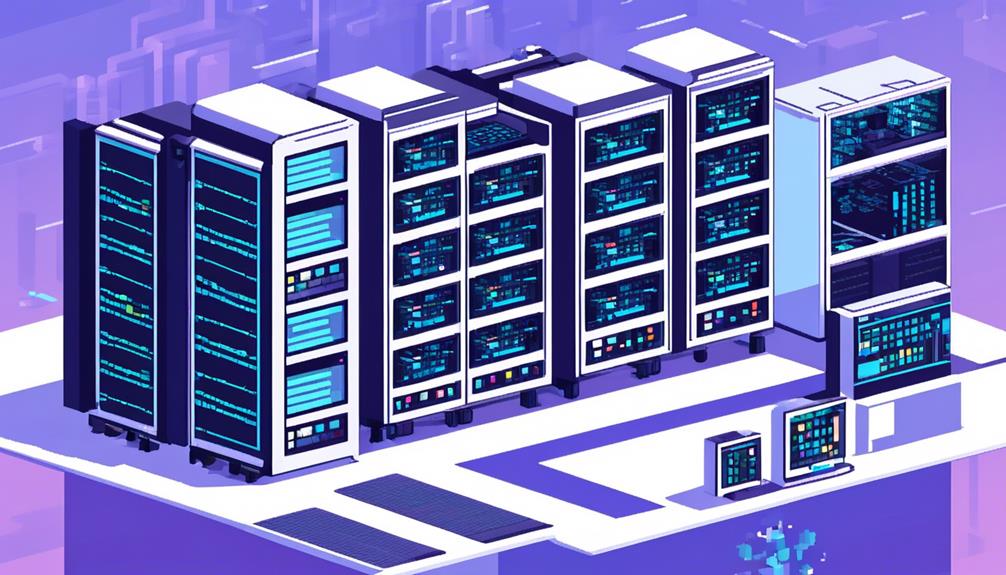
Leveraging AI in edge data centers brings several advantages:
- Real-time analysis: AI enables quick decision-making at the edge, allowing for real-time analysis of data. This capability is crucial in applications where immediate action is required.
- Reduced latency: By processing data locally at the edge, AI in edge data centers reduces latency. This means that the time it takes for data to travel from the source to the data center and back is significantly reduced, resulting in faster response times.
- Improved bandwidth efficiency: Local processing also improves bandwidth efficiency. Instead of sending large amounts of data to a centralized data center for analysis, only relevant information is transmitted. This reduces the strain on network bandwidth and ensures more efficient data processing.
- Optimized infrastructure: Edge data centers can be optimized to meet the specific needs of AI applications. By implementing energy-efficient designs and technologies, these centers can lower power and cooling requirements. This is particularly beneficial for hosting AI applications, which often require substantial computing power.
AI for Real-Time Analysis
Real-Time AI Analysis in Edge Data Centers enables rapid processing and analysis of data at the source. This facilitates real-time decision-making and inference for low-latency AI applications and enhances security, reliability, and scalability for AI deployments.
By leveraging AI in edge data centers, organizations can reduce the volume of data transmitted over networks by processing data locally. This not only minimizes network congestion but also reduces power consumption associated with transferring large amounts of data.
Furthermore, edge data centers with AI capabilities support real-time analysis for IoT devices and industrial automation. This allows for immediate responses and actions based on the data generated. This capability enhances the efficiency and effectiveness of AI applications in various industries, including healthcare, manufacturing, and transportation.
Edge Data Center Benefits
The utilization of AI in edge data centers provides significant benefits in terms of improved efficiency, reduced latency, enhanced security, and independent operation.
Edge data centers enable real-time processing and analysis of data closer to the data source, reducing latency for applications such as IoT, AI, and video streaming. By bringing computing resources closer to the edge, these centers improve bandwidth efficiency by minimizing data transmission over networks.
Additionally, edge data centers offer enhanced security and privacy by minimizing the amount of data sent over long-distance networks, reducing the risk of data breaches. Moreover, these centers provide reliability and independent operation, ensuring continuous application performance even in the event of network disruptions.
Optimizing Edge Infrastructure
With the aim of optimizing edge infrastructure, the integration of AI capabilities in edge data centers revolutionizes the efficiency, scalability, and security of operations.
Here are three ways in which AI is leveraged to optimize edge infrastructure:
- Enhanced Processing Power: AI algorithms enable edge data centers to process and analyze vast amounts of data in real-time, leading to faster decision-making and improved performance.
- Increased Storage Capacity: AI-driven edge infrastructure solutions leverage advanced storage technologies such as distributed storage and intelligent caching, enabling data centers to efficiently store and retrieve data at the edge.
- Improved Performance: AI algorithms can optimize resource allocation, workload distribution, and network management, leading to enhanced performance and reduced latency in edge computing environments.
The Future of AI in Data Center Infrastructure
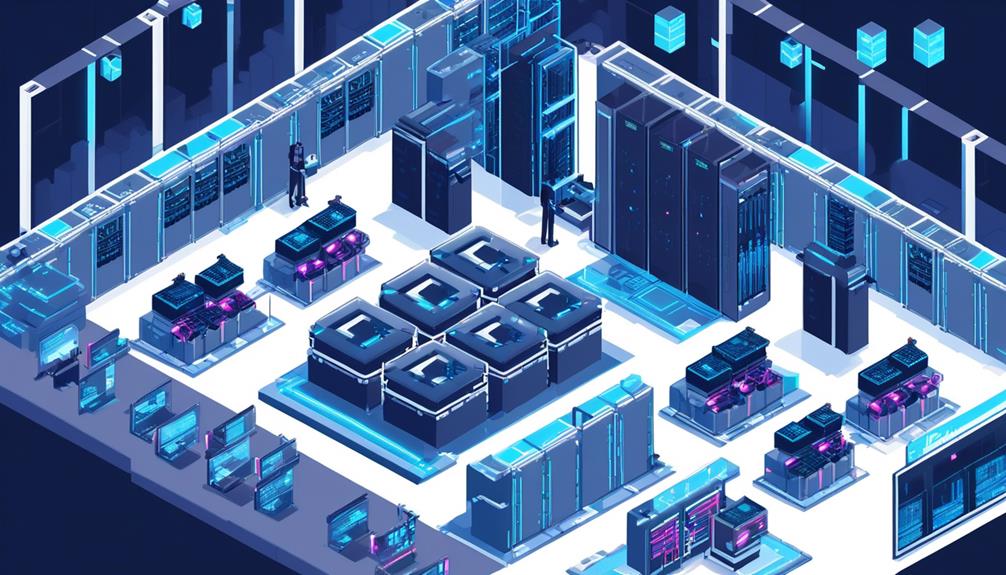
AI data center investments are shaping the future of data center infrastructure by driving advancements in artificial intelligence technology. These investments focus on improving the core infrastructure components of data centers, including servers, storage systems, and networking equipment. By optimizing these elements, AI data centers can achieve faster data processing, improved AI model performance, and streamlined application deployment.
One of the future trends in AI data center investments is the increasing focus on edge computing. Edge computing brings data processing closer to the source, reducing latency and improving real-time analytics. This is particularly important for AI applications that require immediate decision-making, such as autonomous vehicles or industrial automation. By deploying AI capabilities at the edge, data centers can ensure faster response times and enhanced user experiences.
Another future direction for AI data center infrastructure is the integration of AI and 5G technology. The combination of AI and 5G can enable new use cases and applications that require high-speed, low-latency connectivity. This integration will further enhance the capabilities of AI in data centers, opening up possibilities for smart cities, IoT devices, and immersive experiences.
As AI technology continues to evolve, data centers must adapt their infrastructure to keep pace. This means investing in scalable solutions that can handle the increasing volume and complexity of AI workloads. Power consumption is another challenge that needs to be addressed, as AI systems can be resource-intensive. Data centers need to find ways to optimize energy usage while maintaining high performance.
Challenges and Considerations in Implementing Edge Computing and AI
As the landscape of AI data center investments continues to evolve, addressing the challenges and considerations in implementing edge computing and AI becomes crucial. The rise of IoT devices and the demand for edge computing resources have created the need for a distributed computing architecture that brings computation closer to the data source.
Here are three key challenges and considerations in implementing edge computing and AI:
- Reducing latency: One of the main drivers for edge computing is the need to reduce latency. Central data centers often struggle to provide real-time responses due to the distance between the data source and the computing resources. By bringing AI capabilities closer to the edge, organizations can reduce the time it takes to process data and make decisions, enabling faster response times.
- Power consumption and cooling: Edge computing and AI deployments require significant power and cooling resources. As edge infrastructure expands to support AI workloads, it becomes essential to design energy-efficient solutions that can handle the increased power demands. Additionally, effective cooling mechanisms must be in place to prevent overheating and ensure optimal performance.
- Managing distributed systems: Implementing edge computing and AI introduces complexities in managing distributed systems. Organizations need to consider how to efficiently deploy, update, and manage AI models at the edge. This includes addressing challenges such as version control, data synchronization, and security across the distributed infrastructure.
Addressing these challenges and considerations is crucial for the successful implementation of edge computing and AI. As the demand for real-time and localized AI processing continues to grow, organizations must ensure they have the necessary infrastructure and strategies in place to effectively leverage the power of edge AI.
Case Studies Showcasing the Success of AI in Data Centers
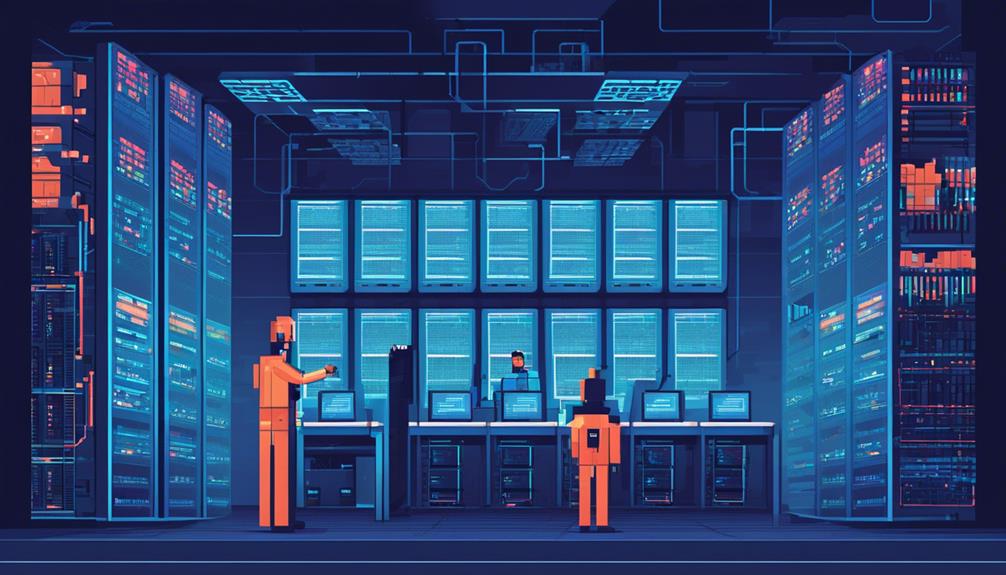
Several case studies demonstrate the successful implementation of AI in data centers, showcasing the transformative power of this technology in enhancing data processing and decision-making capabilities. These case studies highlight the benefits of AI data center investments, such as faster and more accurate data processing, real-time AI applications, and improved model performance. Let's take a look at some contextually relevant examples:
Case Study | Description |
---|---|
Google DeepMind | Google DeepMind implemented AI in their data centers to optimize energy usage. By using machine learning algorithms, they were able to reduce power consumption by 15%, resulting in significant cost savings. Additionally, AI helped improve cooling efficiency, leading to better thermal management and reduced environmental impact. |
Facebook leveraged AI in their data centers to enhance server performance and reliability. Through predictive analytics and machine learning algorithms, they were able to proactively identify and rectify potential hardware failures, minimizing downtime and improving overall system availability. This resulted in improved user experience and increased customer satisfaction. | |
Microsoft | Microsoft utilized AI in their data centers to enhance data security. By implementing advanced AI-based threat detection systems, they were able to identify and mitigate potential cybersecurity threats in real-time. This proactive approach significantly strengthened their security posture and protected sensitive data from malicious attacks. |
IBM | IBM employed AI in their data centers to optimize workload allocation. Through intelligent workload management systems, they were able to dynamically allocate computing resources based on demand, ensuring efficient utilization and reducing operational costs. This resulted in improved resource allocation and enhanced overall system performance. |
These case studies highlight the success of AI in data centers and demonstrate its ability to address various challenges and deliver tangible benefits. As AI technology continues to evolve, we can expect further advancements in edge computing, 5G integration, and other areas, paving the way for even more transformative applications in data centers.
Frequently Asked Questions
Does Edge Computing Use Ai?
Yes, edge computing leverages artificial intelligence (AI) to enable real-time decision-making and innovation. Machine learning plays a crucial role in edge computing by processing and analyzing data closer to its source, reducing latency and enhancing efficiency.
However, implementing AI in edge computing presents challenges such as limited computational power and constrained resources.
The benefits of combining AI and edge computing include reduced latency, enhanced efficiency, and energy savings.
Use cases of AI-powered edge computing include autonomous vehicles, smart cities, and industrial IoT.
The future holds promising possibilities for AI in edge computing, enabling even more advanced real-time decision-making capabilities.
How Does Edge Computing Affect Data Center?
Edge computing has a significant impact on data centers in several ways.
Firstly, it reduces latency by bringing AI processing closer to the data source, enabling real-time decision-making.
Secondly, it requires a different architecture that includes edge infrastructure and hardware.
Thirdly, scalability challenges arise due to the distributed nature of edge computing.
Fourthly, edge to cloud integration becomes crucial to ensure seamless data flow between edge devices and central data centers.
Additionally, data security considerations become critical as data is processed and stored at the edge.
How Is AI Used in Data Centers?
AI is extensively used in data centers to enhance operations and improve efficiency. AI applications in data centers include:
- AI-driven automation, which automates repetitive tasks and reduces human intervention.
- Predictive maintenance, where AI analyzes data to identify potential system failures before they occur.
- AI-powered resource optimization, which optimizes resource allocation in data centers.
- Anomaly detection, where AI is used to identify and address abnormal behavior in the data center environment.
- AI-driven data analysis, enabling quick and accurate analysis of large datasets, providing valuable insights for decision-making.
How Is Edge Computing Different From Data Centers?
Edge computing and data centers have distinct differences in terms of infrastructure and functions.
Edge computing involves processing data closer to the source, enabling real-time decision-making and reducing latency.
In contrast, data centers centralize data processing and storage, allowing for large-scale data operations.
Edge computing offers advantages such as reduced network traffic and localized processing.
However, implementing edge computing poses challenges like limited resources and security risks.
Despite these challenges, edge computing is expected to play a pivotal role in the future, complementing data centers and driving advancements in localized processing and efficiency.